Import Libraries
from sklearn.model_selection import train_test_split
from sklearn.ensemble import RandomForestClassifier
from sklearn import preprocessing
from sklearn.metrics import accuracy_score
from sklearn.metrics import classification_report
from sklearn.tree import export_graphviz
from sklearn.metrics import f1_score
import matplotlib.pyplot as plt
from subprocess import call
import numpy as np
import pandas as pd
Read Dataset
data = pd.read_csv('winequality-red.csv')
data.describe()
Output:

Selecting Features and target variable
x = data.drop('quality', axis=1)
y = data['quality']
Split the dataset
X_train, X_test, y_train, y_test = train_test_split(x, y,
test_size=0.2,
random_state=123,
stratify=y)
Normalize the Dataset
scaler = preprocessing.StandardScaler().fit(X_train)
X_train_scaled = scaler.transform(X_train)
X_test_scaled = scaler.transform(X_test)
Fit Into the model
forest = RandomForestClassifier(n_estimators=100,
bootstrap = True,
max_features = 10,
n_jobs = 1,
criterion = 'entropy')
forest.fit(X_train_scaled, y_train)
Output:
RandomForestClassifier(bootstrap=True, class_weight=None, criterion='entropy', max_depth=None, max_features=10, max_leaf_nodes=None, min_impurity_decrease=0.0, min_impurity_split=None, min_samples_leaf=1, min_samples_split=2, min_weight_fraction_leaf=0.0, n_estimators=100, n_jobs=1, oob_score=False, random_state=None, verbose=0, warm_start=False)
Mean Absolute Error
pred = forest.predict(X_test_scaled)
errors = abs(pred - y_test)
print('Mean Absolute Error:', round(np.mean(errors), 2))
Output:
Mean Absolute Error: 0.34
Accuracy Score
print("Random Forest Accuracy Score -> ",accuracy_score(pred, y_test)*100)
print(classification_report(y_test, pred))
print(f1_score(y_test, pred , average='weighted'))
Output:
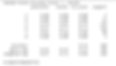
Visualize
col = x.columns
y = forest.feature_importances_
fig, ax = plt.subplots()
width = 0.4 # the width of the bars
ind = np.arange(len(y)) # the x locations for the groups
ax.barh(ind, y, width, color="green")
ax.set_yticks(ind+width/10)
ax.set_yticklabels(col, minor=False)
plt.title('Feature importance in RandomForest Classifier')
plt.xlabel('Relative importance')
plt.ylabel('feature')
plt.figure(figsize=(5,5))
fig.set_size_inches(6.5, 4.5, forward=True)
Output:

Are you looking Machine Learning Assignment Help? Big Data Assignment Help? Or any other Python related help?
Send Your requirement details at:
And get instant help with an affordable price.