Requirement Details
An organization wanted to check association among employee experience, skills, traits etc. to better manage human resources.
As a data scientist, you are required to recognize patterns from the available data and evaluate efficacy of methods to obtain patterns. Your activities should include - performing various activities pertaining to the data such as, preparing the dataset for analysis; investigating the relationships in the data set with visualization; identify frequent patterns; formulate association rules and evaluate quality of rules.
Demonstrate KDD process with following activities:
Problem statement
·Perform exploratory data analysis
Preprocess the data
Propose parameters such as support, confidence etc.
Discover frequent patterns
Iterate previous steps by varying parameters
Formulate association rules
Compare association rules
Briefly explain importance of discovered rules
Following are some points for you to take note of, while doing the assignment:
The data in some of the rows in the data set may be noisy
Some of the attributes have large number of values – you can consider merging them into 2 or 3 values to simplify the solution
State all your assumptions clearly
Provide clear explanations to explain your stand
Solution:
Import Libraries
#import libraires
import pandas as pd
from sklearn.model_selection import train_test_split
from sklearn.cluster import KMeans
from sklearn.metrics import mean_absolute_error, make_scorer
import sklearn.metrics as metrics
import matplotlib.pyplot as plt
from sklearn.metrics import confusion_matrix
import seaborn as sns
import itertools
import numpy as np
import matplotlib.pyplot as plt
Read Data
#Read Dataset
df_train = pd.read_csv("Employee_skills_traits.csv")
df_train
Output:

Replace column attribute space
#Replace Column attribute space with '-'
df_train.columns = df_train.columns.str.replace(' ','_')
Now Check columns
#Show all dataset Columns
df_train.columns
Output:
Index(['ID', 'Employment_period_', 'Time_in_current_department_', 'Gender_',
'Team_leader_', 'Age_', 'Member_of_professional_organizations_',
'.Net_', 'SQL_Server_', 'HTML_CSS_Java_Script_', 'PHP_mySQL_',
'Fast_working', 'Awards', 'Communicative_'],
dtype='object')
Check Data After Rename Attribute
df_train

Here we see that all column name are renames and space is removed(Here we do this steps because space raise issue to read columns)
Checking Dataset Null Values
#Checking Null Value
#Visualize for check null value
check_null_value = df_train.isnull()
sns.heatmap(check_null_value,yticklabels=False,cbar=False,cmap='viridis')
Output:

As per above heat map we can say that it has no null value.
Checking Shape of dataset
#– the shape of the dataset
df_train.shape
Output:
(998, 14)
Dataset has 998 rows and 14 columns
Checking Data Type
#– info of the dataset
df_train.info()
Output:

Summary of the dataset
#– summary of the dataset
df_train.describe()
Output:

Finding Features and target variable and Split dataset
#Devided dataset into with target attribute "Awards"
x=df_train.drop(['Awards'],axis=1)
target=df_train.Awards
#split dataset with 25 percent test sample
X_train, X_test, y_train, y_test = train_test_split(x, target, test_size=0.25, random_state=0)
Use K means Clustering Algorithm And Fit into Model
#Use Data mining Clustering and Find the Mean Square Error
kmeans = KMeans(n_clusters=2)
kmeans.fit(X_train, y_train)
y_pred = kmeans.predict(X_test)
test_mae = mean_absolute_error(y_test, y_pred)
print('Validation MAE: %0.3f' % test_mae)
Output:
Validation MAE: 0.548
Accuracy
#Find Accuracy of Model
score = metrics.accuracy_score(y_test,y_pred)
print('Accuracy: %0.2f' % score)
Output:
Accuracy: 0.45
Fine Tune the Model To increase the accuracy
#Use Data mining Clustering and Find the Mean Square Error
#Fine Tune The Model to minimize the MSE and increase Accuracy
kmeans = KMeans(n_clusters=2, init='k-means++', n_init=5, max_iter=100, tol=0.0001)
kmeans.fit(X_train, y_train)
y_pred = kmeans.predict(X_test)
test_mae = mean_absolute_error(y_test, y_pred)
print('Validation MAE: %0.3f' % test_mae)
Output:
Validation MAE: 0.452
Accuracy
#Find Accuracy of Model
#Find Accuracy After Fine tune Parameter
score = metrics.accuracy_score(y_test,y_pred)
print('Accuracy: %0.2f' % score)
Here we have see increase the model Accuracy when we have tune the model using extra K means parameters
Plot the Confusion Matrix
#Evaluation of Model - Confusion Matrix Plot
def plot_confusion_matrix(cm, classes,
normalize=False,
title='Confusion matrix',
cmap=plt.cm.Blues):
"""
This function prints and plots the confusion matrix.
Normalization can be applied by setting `normalize=True`.
"""
if normalize:
cm = cm.astype('float') / cm.sum(axis=1)[:, np.newaxis]
print("Normalized confusion matrix")
else:
print('Confusion matrix, without normalization')
print(cm)
plt.imshow(cm, interpolation='nearest', cmap=cmap)
plt.title(title)
plt.colorbar()
tick_marks = np.arange(len(classes))
plt.xticks(tick_marks, classes, rotation=45)
plt.yticks(tick_marks, classes)
fmt = '.2f' if normalize else 'd'
thresh = cm.max() / 2.
for i, j in itertools.product(range(cm.shape[0]), range(cm.shape[1])):
plt.text(j, i, format(cm[i, j], fmt),
horizontalalignment="center",
color="white" if cm[i, j] > thresh else "black")
plt.ylabel('True label')
plt.xlabel('Predicted label')
plt.tight_layout()
# Compute confusion matrix
cnf_matrix = confusion_matrix(y_test, y_pred)
np.set_printoptions(precision=2)
# Plot non-normalized confusion matrix
plt.figure()
plot_confusion_matrix(cnf_matrix, classes=['0','1'],
title='Confusion matrix, without normalization')
Output:

Find Recall And Precision
#Find Recall and Precision
cnf_matrix = confusion_matrix(y_test, y_pred)
recall = np.diag(cnf_matrix) / np.sum(cnf_matrix, axis = 1)
precision = np.diag(cnf_matrix) / np.sum(cnf_matrix, axis = 0)
Confusion Matrix:
cnf_matrix
Output:
array([[63, 61],
[52, 74]], dtype=int64)
Precision:
#Precision Score
precision
Output:
array([0.55, 0.55])
Recall Score:
#Recall Score
recall
Output:
array([0.51, 0.59])
Exploratory Data Analysis
#EDA(Exploratory Data Analysis)
#ploting Graph of co-rrelation
fig = plt.figure(figsize=(10,5))
sns.heatmap(df_train.corr())
Output:

Box Plot
#Box Plot between ID & Employment_Period
#Here Both attribute(ID & Employment_period_) is associated to each other
sns.boxplot(x=df_train['Employment_period_'],y=df_train['ID'] )
Output:
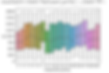
# visualize frequency distribution of `Gender` variable
f, ax = plt.subplots(figsize=(9, 7))
ax = sns.countplot(x="Gender_", data=df_train, palette="Set1")
ax.set_title("Frequency distribution of Gender variable")
ax.set_xticklabels(df_train.Gender_.value_counts().index, rotation=30)
plt.show()
Output:

Line Chart
#Bar Plot(Here we choose some records of daset due to show visible plots)
df_train_plot = df_train.iloc[:18]
df_train_plot.plot(x= "ID", y= "Employment_period_", kind="bar")
Output:
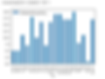
If you need any other help related to machine learning then send your requirement details at realcode4you@gmail.com and get instant help.